Shingirai’s upcoming talk on the use of machine learning tools to classify AGN host galaxy morphologies in large JWST surveys at the 32nd International Astronomical Union General Assembly 2024
Shingirai is a first year PhD student at Lancaster University in the UK where he is supervised by Dr. Brooke Simmons. Before his PhD, he completed his BSc at Aberystwyth University in Wales, where with the assistance of a Peter Hancock scholarship, he achieved a first class honours degree. Combined with undergraduate research experience at the University of Oxford, CEA Paris-Saclay, and his undergraduate University of Aberystwyth, he has a had a variety of research experiences at his young academic age.
Shingirai is also the Vice-president of the Zimbabwean Astronomical Society which he is co-founding with the ZAS President Thobekile, and that is being run with the assistance of many other members from Zimbabwe. His is passionate and active in astronomical outreach, and he hopes to realise ZAS’ aim of Zimbabwe eventually reaching capabilities to produce professional astronomical research within the country.
The talk that Shingirai will be presenting will be titled “Deep Learning Classifications of AGN Host Galaxies in Large JWST Surveys”
Highly Obscured AGN have unique visual identifiers in new JWST surveys that may allow them to be reliably detected in methods completely independent to other detection methods. Combining this knowledge with powerful tools like Zoobot, and Galaxy Zoo volunteer classifications, there are many interesting findings to present.
Title:
Deep-learning classifications of galaxy morphologies in large JWST surveys.
Abstract:
Investigations of AGN host galaxies including their merger history and morphology have been conducted up to z ~ 2. However, new JWST surveys such as COSMOS-Web and CEERS permit investigation of the properties of AGN host galaxy populations in rest-frame optical between 0.5 < z < 4; this spans the entire cosmic noon period where both SMBH growth and galaxy star formation rates peak. We use the deep-learning classifier ‘Zoobot’ to determine galaxy morphologies in the aforementioned surveys. This is conducted via a transfer learning method that inherits prior training of Zoobot on ground-based imagery. Predictions of the JWST morphologies are then applied to the large multi-wavelength imaging set of COSMOS-Web, after being fine-tuned using Galaxy Zoo volunteer classifications of CEERS images. We further refine the AGN host galaxy classifications by training Zoobot to ignore the central AGN; we then assess the effectiveness of this method before comparing the evolution of host galaxy morphologies with that of inactive galaxies during this era of galaxy assembly. During this talk, a sample of identified AGN host galaxies will be presented, as well as a control sample of inactive galaxies that have been matched on properties such as stellar mass and redshift. Initial results on the differences between morphologies of AGN hosts, and their inactive counterparts will be shown.
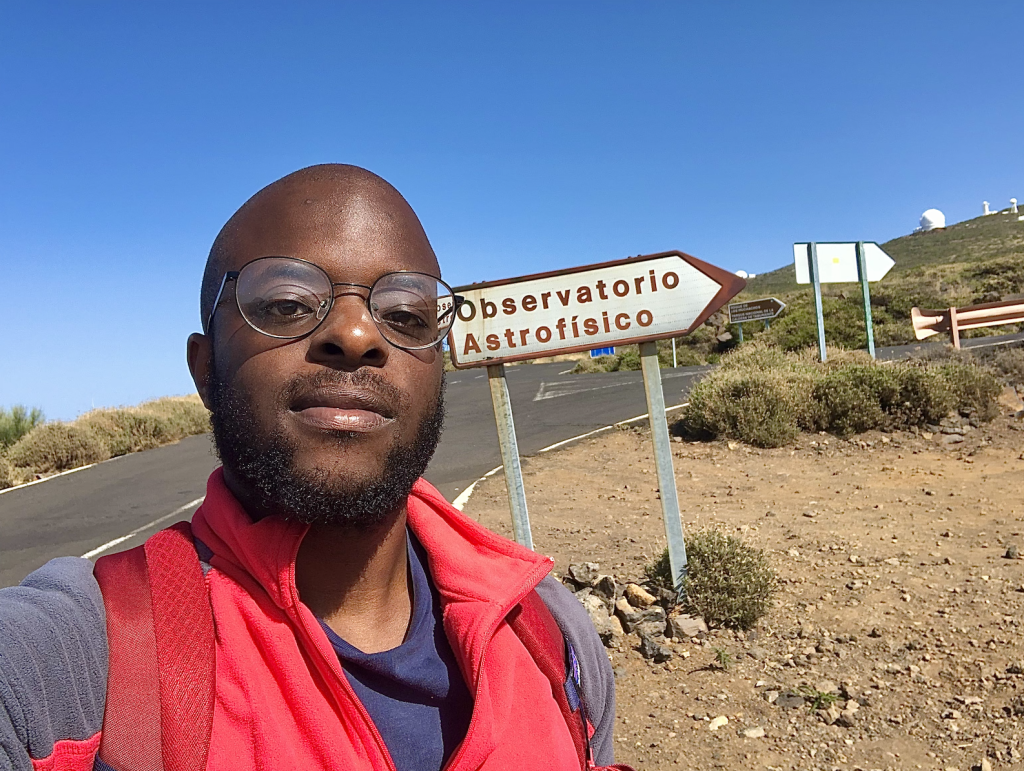